The KARLI PROJECT
Call for funding: ‘Artificial intelligence as a key technology for the vehicle of the future’.
KARLI: Artificial Intelligence for an adaptive, responsive and level conform interaction with future vehicles.
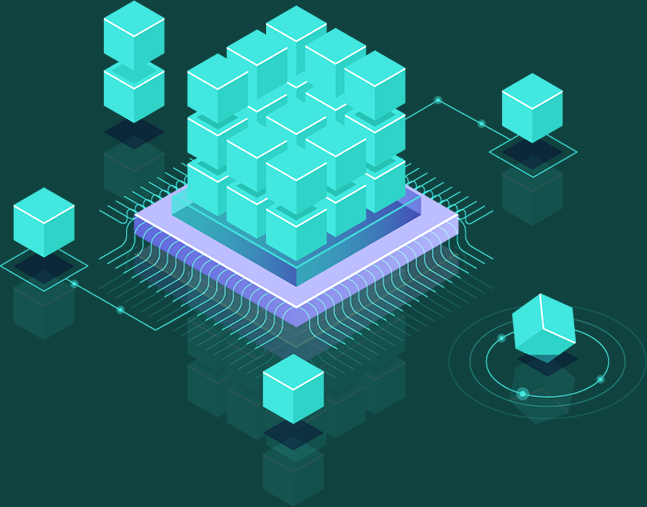
At a glance
Overall objective of the project
KARLI’s goal is to develop an adaptive, responsive and level-compliant interaction in the vehicles of the future.
For this purpose, customer-relevant AI functions are developed in KARLI that capture driver states and shape interactions for different automation levels. These AI functions are developed in KARLI from empirical and synthetically generated data. The data will be collected and used in KARLI in such a way that the project results are scalable to Big Data from production vehicles available in the future. KARLI provides the specifications and requirements for the vehicle architecture and sensor technology of the future so that upcoming interior sensors collect optimal Big Data for driver-vehicle models and AI interactions.
The research guiding hypothesis is that AI-based driver-vehicle state models will achieve the necessary goodness and robustness to enable multilevel automated vehicles through situationally adaptive human-machine interactions (HMI).
The consortium assumes that vehicles that can handle multiple levels of automation will not be ready for the market if the level-specific requirements for occupants and drivers are not mapped in a dedicated way in the HMI. The connection between adaptive and also personalized HMI and level-compliant driver state recognition leads to KARLI’s goal of defining, recognizing, and encouraging level-compliant behavior.
The driver-vehicle state models (DVSM) developed in KARLI will be continuously enhanced by future Big Data from the vehicle interior, and KARLI can define the requirements for this Big Data based on Small2Big data collection (see explanation below) even before the Big Data is collected in series production.
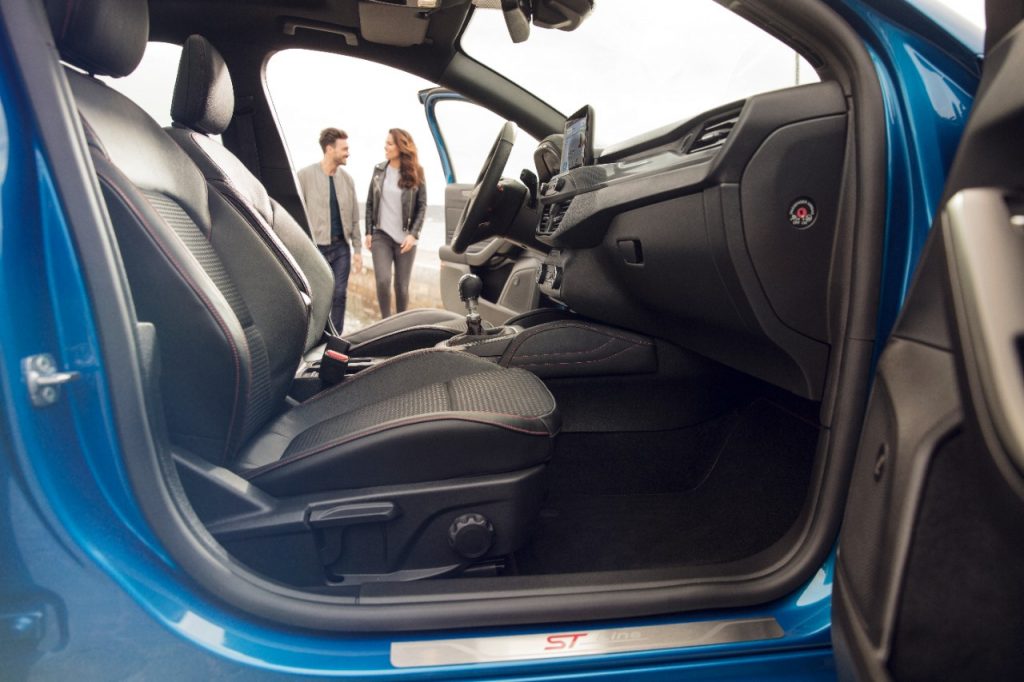
This approach improves the data quality that future vehicles provide for DVSM and HMI. Another prerequisite for the deployment of AI-based DVSM and HMI is AI assurance, e.g., with respect to the robustness of AI-DVSM and the prevention of misuse and malicious attacks on the AI-HMI. KARLI tests different methods for this purpose and evaluates, among other things, their influence on the measurable user experience of the overall system. The development of UX design dimensions, guidelines, and test methods specifically for AI-HMI are also aimed at this.
The particular challenge in implementing the project objective …
… is the large variance of possible vehicle interiors. First, AI approaches, e.g. from IncarIn, PaKOS (Fraunhofer) and IFAS (Continental) successfully used a controlled, recorded, and manually labeled camera image dataset with limited state space.
However, the AI-models based on this are limited to the label in the data set. The KARLI approach investigates how AI-methods can be better trained for real-world applications using less structured, but large and multisensory data sets (Big Data). The approach is to first use a relatively small, manually labeled data set, but then to steadily expand it during the project with less labeled or completely unlabeled data from real situations. This approach is subsequently called the “Small2Big Data” approach.
In the project, data collection will primarily involve real drives to ensure the transferability of the results (Jipp & Schnieder, 2020). These are supplemented by synthetically generated data. KARLI thus addresses the high demand for empirical AI-training data and investigates how such data can be profitably used in AI procedures. Approaches include AI-assisted Manuel Labeling, Transfer Learning, Weakly Supervised Learning, or Zero Shot Learning. The appropriate methods will be selected in the concept phase of the project and compared during the project lifetime. The selected methods will also allow the later use of data from production vehicles to further increase quality, capture edge cases, and adapt the methods to changing behaviors.
Due to the complexity and multitude of variables that need to be captured, not only indoors, only AI approaches can meaningfully interpret the expected amount of multidimensional data and achieve significant advances in robustness and situational fit (Ludwig et al., 2018).
Adaptive interactions are also only technically feasible through the use of machine learning. KARLI takes up this challenge and sets the foundation for the integration of AI-based, adaptive, responsive and level-compliant human-machine interactions in the intelligent vehicle “Made in Germany”.
The target applications of KARLI …
… are aligned with the overall goal of “level-compliant driver behavior”. The current driving situation, including the automation level, results in concrete requirements for the driver’s state, his behavior and the predictable ability to act. The recording of the driving situation and the driver’s state thus allows an actual-goal comparison and a targeted approach in the dialog between man and machine. Thus, on the one hand, mode and situation awareness on the part of the driver is created and the readiness for transition is promoted, and on the other hand, the trajectory planning and the automation level are adapted to the driver’s state. KARLI promotes driving with the highest possible level of automation, which has a positive effect on efficiency and safety. At the same time, motion sickness, misuse and abuse of the automation function are detected and avoided.
The detection of the driver’s state and the driving situation as well as the adaptive MMI are realized by using AI technologies. For this purpose, AI-optimized data collection is performed in relevant driving scenarios on the road. The Small2Big Data approach and the generation of synthetic training data allow the scalability of the AI methods to future Big Data from production vehicles. The project result is an adaptive, responsive and level-compliant in-vehicle interaction consisting of AI-based DVSM and HMI. These will be developed in the Small2Big Data approach with new AI fusion models and piloted and evaluated in real vehicles.
Figures and data
Facts about the KARLI project
01. July 2021 – 30. June 2024
TÜV Rheinland Consulting GmbH
Federal Ministry for Economic Affairs and Climate Action
Heiko Schnieders, Continental Automotive GmbH
Dr. Frederik Diederichs, Fraunhofer IOSB
Continental, Ford, Audi, INVENSITY, paragon semvox, TWT, studiokurbos, Fraunhofer IAO, Fraunhofer IOSB, allround team, Hochschule der Medien, Universität Stuttgart, branmatt || legal
15.6
million € project volume
9.7
million € project funding
36
months project duration
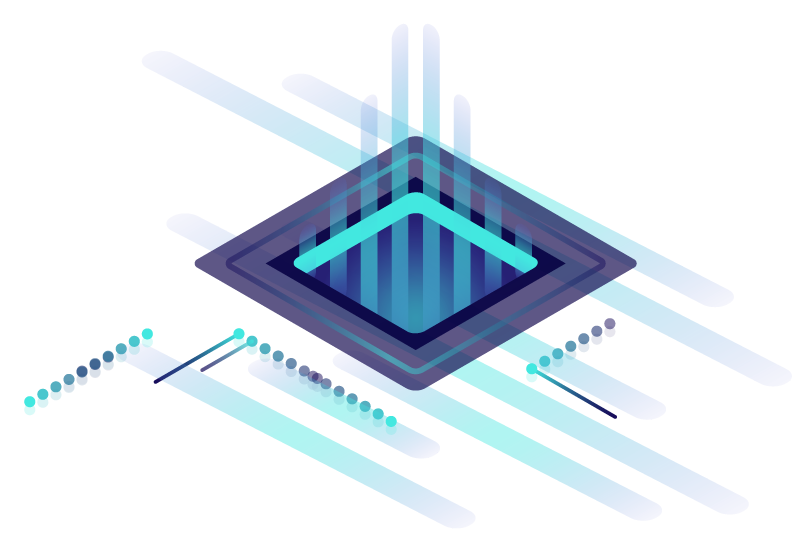